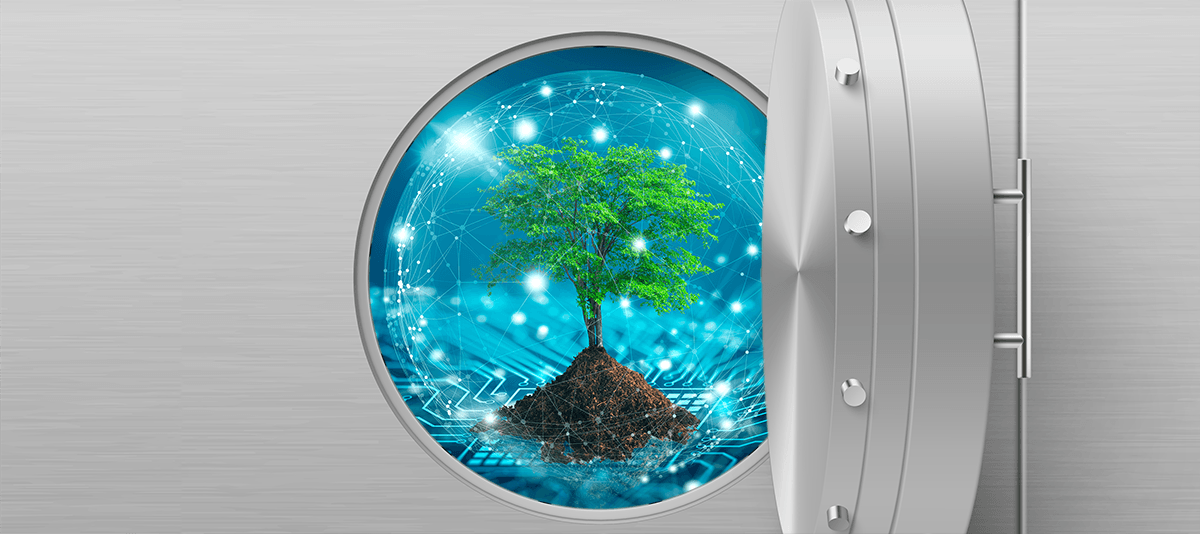
Environmental Data Space: Incorporating AI and Machine Learning
How Innovation Fits into a Green Future
Humanity can achieve great things when we’re all working together, sharing our knowledge and information to build a better world.
In our day and age, that sharing often takes the form of data sharing.
So-called Open Data is shared with anybody, with very few limitations in place. Such sharing of data has many advantages, for instance in science and education. It enables people of different backgrounds and varying expertise to access the same source material. The more specialists can use the same data to create, build upon, and verify experiments, the faster vital breakthroughs can occur.
Artificial Intelligence (AI) solutions are also only as effective as the data they are trained upon allows them to be. Even the greatest Machine Learning algorithm needs a basis of strong data to learn from before it can provide useful results.
However, not all data can be shared as Open Data.
Noise data around a factory can indicate the levels of activity going on there to competitors, so sharing it openly can have disadvantages for the owner. Geodata that indicates the exact locations of protected flora and fauna can, in the wrong hands, do more harm than good for environmental protection. Data associated with natural persons may fall under the GDPR.
Fortunately, data sharing needn’t be “all or nothing”.
A solution that balances the legitimate interests of data users and the data sovereignty of data providers and subjects exists.
In this article, we explain and explore that solution.
Welcome to data spaces.
So, what is a data space?
Most of you will have heard of digital platforms and the winner-takes-all patterns they tend to fall in. In such a monolithic platform, there are huge hurdles to innovations that are not pursued by the platform owner. Prominent examples are current social networks that exploit users and user-generated data, often beyond the control of the originator of the content.
Innovation is hampered by this model. Consider that social networks primarily get revenue from selling ads. The networks are therefore primarily incentivised to sell ads and generate more revenue, as opposed to focusing on technological breakthroughs that would have wider implications.
Compared to that, a data space is a type of collaboration model that utilises a decentralized infrastructure. This means that data is not stored or controlled centrally, but at the sources. There is no central repository or platform into which data owners supply their data and from which consumers access and retrieve data. Instead, data is exchanged directly between appropriate parties. To ensure only trusted data sharing and exchange in data ecosystems can take place, the data space bases access to data on commonly agreed principles.
Data spaces facilitate the secure exchange, linkage, and interoperability of data within an ecosystem. They do so by using data standards and collaborative governance models. This preserves the digital sovereignty of data owners and subjects, whilst still providing those with proven legitimate interests a level of accessibility to data that would otherwise not be available at all.
Demands surrounding sensitive data can include:
- Protection of business interests from competition in industrial settings
- Protection of personal data, such as when accessing health services
In either case, opportunities for innovation would benefit from the seamless exchange of data with trusted partners. For this, the decentralized data space approach allows all participants to retain digital sovereignty. Data can remain with the owners until it is retrieved under controlled conditions.
To meet the fundamental requirements of a data space in terms of trust between participants, data security, and interoperability, a federator needs to be set up. The responsibilities of this federator include, among others responsibilities, cataloguing and brokering data sources.
Architecture of a Data Space
There are different approaches to defining the architecture of data spaces. For clarity, the International Data Spaces Association (IDSA) has defined the IDS-RAM (reference architecture model). This cross-sectoral model is characterized by an open, reliable and federated architecture for cross-sectoral data exchange. It contains a basic set of components necessary to build a robust data space.
These components are:
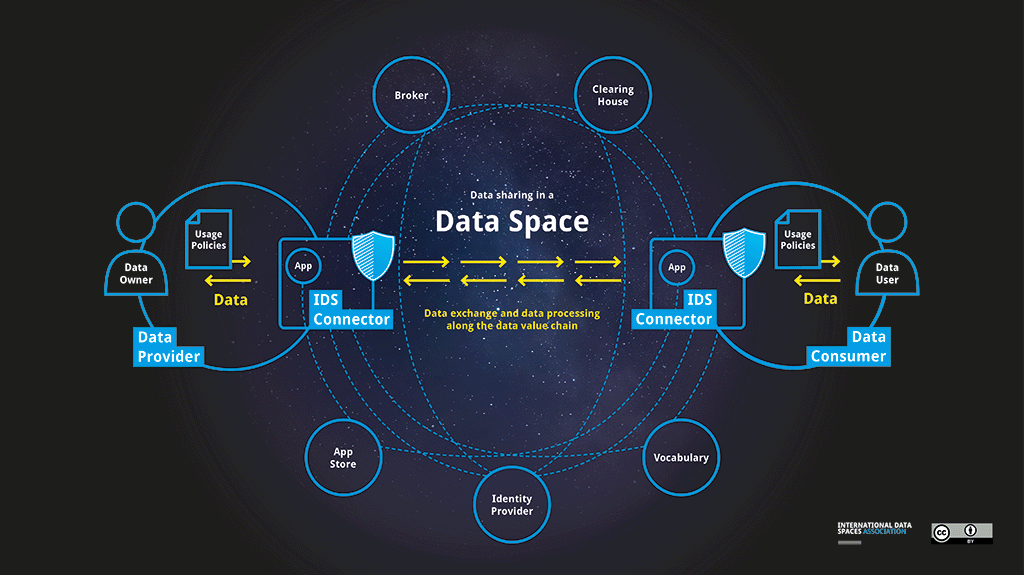
Image Source: IDSA
Connectors
The connector is the central technical component for secure and trusted data exchange, through which participants provide and access data in a data space. This component handles the data according to policies defined by the data owner in terms of access and usage rights, thus ensuring its sovereignty.
Such connectors publish the description of their data endpoints with a metadata broker (see below). This allows potential data consumers to look up available data sources and data in terms of content, structure quality, actuality and other attributes. Connectors can be certified, e.g. through the IDSA certification programme, in order to prevent malfunction and to guarantee their integrity and compliance.
- Metadata Brokers
The metadata broker is a catalogue then enables data consumers to find assets that have previously been published through a connector into the data space. The metadata in the catalogue follows the generic connector architecture described in the reference architecture model.
- Vocabulary Providers
A vocabulary provides the shared terms according to which data is systematically organised, categorised, and labelled in the data space. Such a shared vocabulary is essential for interoperability. Vocabulary providers manage and offer vocabularies and ontologies, reference data models and metadata to annotate and classify data sets, describe the data sets’ relationships, and define possible constraints.
- Identity providers
An identity provider creates, maintains, manages, and validates identity information for clients and also provides authentication services for trusted applications within a federated or distributed network.
- App Stores
App stores offer applications approved by the governing bodies of the data space. These can be integrated into connectors to communicate and perform tasks such as data transformation, aggregation, and analysis, or can be deployed as standalone smart services that utilize a connector.
There are applications designed for different purposes. For example, system adapters on the data provider side to interface with external entities might be on offer. On the consumer-side are data applications to handle, among other things, the processing, transformation, and analysis of data.
- Clearing house
A clearing house allows participants to keep control of the operations that are being carried out within the data space. The IDS clearing house, for instance, provides decentralised and auditable traceability of all transactions.
The Environmental Data Space Community
From our perspective, one of the main areas where the concepts of data spaces will make a difference in the future is environment, health and climate. By establishing the Environmental Data Space Community (EDSC), we support the European Green Deal Initiative. Thus, the EDSC helps to leverage the enormous potential of data to support measures in areas such as climate change, circular economy, zero pollution, biodiversity, deforestation, and compliance.
Its core mission is to facilitate applying the standards and principles of the International Data Space Association to environmental data. This will foster the availability of high-quality data for any type of private or public decision-making process that has an impact on the environment.
Nine common European data spaces have been defined thus far:
- Health
- Industrial
- Agricultural
- Financial
- Mobility
- Green Deal
- Energy
- Public Management
- Skills
The objective of an Environmental Data Space, in addition to achieving a true sustainable digital transformation as envisaged in the Green Deal initiative, is to facilitate and address the data-oriented interests of all stakeholders while respecting societal and economic interests.
At the moment, over 90% of all environmental data is classified as “sensitive” and therefore not publicly accessible. This means that authorities, enterprises, and citizens lack critical data when addressing environmental and climate challenges.
Within the framework of a trusted data space, classified and open data can be shared in a secure manner, while retaining full sovereignty over one's data and the usage of that data.
Initiatives pioneering work on the building blocks of the Environmental Data Space include, for instance, the Forestry Data Space, Soil, and Noise Mapping.
The Forestry Data Space - Walk through a real case study
The Forestry Data Space is a concrete example of a data space developed within the EDSC. It is a great case study on the importance and inner workings of the data space approach.
Rapid global warming and associated extreme weather events such as storms, heavy rainfall, and prolonged dry periods, as well as the increased occurrence of insect pests, pose a considerable risk to forestry. For instance, the prolonged drought of recent years has led to massive forest damages in Germany.
To address some of these challenges, the FutureForest R&D project, funded by the BMUV (Federal Ministry for Environment, Nature Conservation and Nuclear Safety), is developing the Forestry Data Space, including a decision support system to assist stakeholders such as forestry managers.
The project includes research on AI-assisted tree species and tree vitality recognition from remote sensing data, as well as the development and deployment of ground-based sensor systems to measure soil moisture, temperature, and tree growth, which can be correlated.
One of the project’s goals is to develop the FF.ai Decision Support System (DSS) for climate-adapted forest conversion. This innovation in environmental management will provide stakeholders with recommendations for forest management specifically catered to their local requirements.
The recommendations are based on an ensemble of climate scenarios and includes forestry and ecological indicators, such as wood increment, carbon storage, and biodiversity. Based on this, the future suitability of tree species for a specific location can be derived, allowing the system to provide stakeholders with intelligent feedback and recommendations.
For all the data exchange between the partners as well as to provide the data for the DSS, the project includes the development of a Forestry Data Space based on the data space standards set by the IDSA. We defined the framework architecture, the services and connectors, the security concept, and the technical implementation of the FDS.
Data sets (climate, temperature, soil, etc.) can be made available in the FDS by the relevant institutions at any level of openness they feel pertinent, be it highly restricted or as fully open data. Additional data, such as elevation, tree species, and condition maps, can be provided by appropriate research institutions and/or service providers.
One of the data gaps in this scenario is the user-generated location-specific data, for example the local stand, tree species, and management methods. These can be provided by forestry stakeholders such as planning authorities, municipalities, and forest owners, who thus fulfil the dual role of data providers and consumers.
When compared to any web- and cloud-based solution currently available in the market, a data space is the only solution where all these data sets can be incorporated in a trusted environment, federated and governed by well-defined rules to maintain data sovereignty.
In addition to the outlined business case of a decision support system, further use cases can be identified. For example:
- generating projections and market trend reports utilising hyperlocal datasets for a wider audience, such as investment managers, real estate agencies, and government bodies
- establishing a trustworthy marketplace for forestry equipment, certified and traceable timber trade, etc. within the FDS
wetransform GmbH is the leading partner of the FutureForest project, in charge of the development of FF.ai DSS and Forestry Data Space. Leveraging many years of experience and know-how in data harmonisation and interoperability involving GIS and environmental data, such as handling the INSPIRE directive and other data transformation to clear and open standards.
We constantly look to collaborate with stakeholders at all levels, from forest practitioners to corporations, research institutions and policy makers.
Should you be interested in a partnership, or want to learn more about how you can contribute to green innovation, reach out to us!