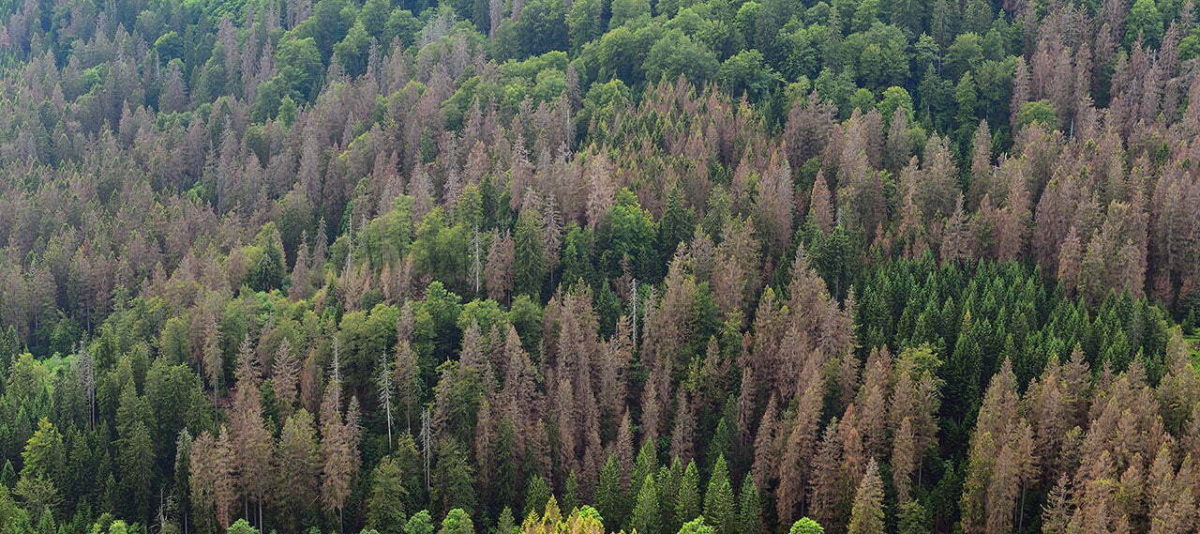
Adapting to Technology: The Evolution of Nature Management
From June 20th to 23rd, 2022 the German “Bundesamt für Naturschutz (BfN)” (Federal Agency for Nature Conservation) organised the workshop ‘AI for Nature Conservation’ on Vilm, a tiny island to the east of the sister island of Rügen.
As I settle into my train seat for my journey home, I think back to the beauty of the island of Vilm. When summer reaches that part of Germany, nature envelops you in the green and blue of forest and sea, the latter often emerging unexpectedly from behind a majestic beech.
There could have been no better place to gather a passionate group of experts from the worlds of technology and environmental management to talk about the best ways to preserve nature through the use of artificial intelligence. It presented me with the perfect opportunity to discuss our Future Forest project with a wider audience, and to learn more about other innovations in environmental technology.
I spend the rest of the ride thinking about what I have learned this week.
Modern Solutions for Modern Problems
During my time on the island, I discovered that there is a lot of excitement and aspiration to use numerical models in order to address the various problems that affect our environment.
This ranges from insect recognition (you can learn more on the websites of kinsecta, BfN, and Julius Kühn-Institut) to classifying wilderness from anthropologically modified areas; to forest conversion; to protecting the seas from plastic pollution; to assessing the risk of natural disasters; just to name a few!
Many advances have also been made in the acquisition and analysis of various types of data: from trap cameras for the classification of insects; to the use of audio sensors for the classification of birds; to metabarcoding: a new method for classifying different vegetative species based on genetic analysis.
A recurring theme during the various presentations and discussions was the need for readily available interoperable data. Data collected in a common collection point that would ensure its access, exchange, and reliability. Something that would improve networking between researchers and practitioners in the field.
That’s how I knew that our Future Forest project was headed in the right direction. The creation of a data space was already designated as one of our project milestones!
In spite of all that ambition and excitement, there were also topics during the workshop that I was more critical of. For example, the desire to exchange models and methods for data analysis. The idea behind this is to allow for easier access to the understanding and use of machine learning models, so they can be used in various fields of research.
I agree that the use and refinement of models by different researchers is certainly the way to confirm and improve results.
Nevertheless, I remain convinced that the two major fields of knowledge involved in this type of projects, namely numerical modelling and knowledge of the specific, physical problem, must remain in the hands of the experts in each individual field.
Research and progress must go forward through teamwork in which the two aspects are dealt with and validated by the respective experts.
Another interesting topic of discussion was the use of efficient and sustainable AI implementations.
When we talk about respecting nature, we cannot ignore the impact made by generating the energy required for calculation and model training. Power consumption is an inevitable factor in this process and we will have to find the right trade-off between the accuracy of the models and their sustainability.
Speaking of trade-offs, that brings me to the trade-off we have to find again between the accuracy of the models and their interpretability.
The key to making final users open to trusting AI is in proposing models that are interpretable and understandable. During the conversation, I often wondered whether this point was made sufficiently clear.
How do we achieve the right level of clarity for those who will use the results of machine learning models in the field? Is what is explicable for a mathematician also explicable for a wider audience?
And how do we guarantee a course of research that brings at the end concrete applicable tools on the table of who is taking practical care of the environment? There is still a very real risk that environmental tech projects run their course without producing a usable tool or other product. Given that these projects are often financed for a finite number of years, introducing Agile development methodologies could be a vital turning point in this.
As mentioned in one of the presentations, Agile methodologies would not only guarantee continuous progress towards a concrete product, but would also introduce a rigorous software implementation framework that ensures efficiency and correctness.
The Road Ahead
As my journey home takes me onto a bustling regional train, I look back through my notes and memories from the week on Vilm to draw my conclusions.
The community of experts I have met on the island is buzzing with potential and, now that our Future Forest project has officially begun, I am eager to start experimenting with the most interesting data and methodologies.
I shall have to choose my cards wisely. At the end of the three-year funding period, we want to deliver a solid proof-of-concept to the community; a tool - perhaps still to be improved - but one that can concretely help forest managers arm forests against climate change.
We need to understand who our users will be and what kind of help we can provide to them.
We need to start collecting the data we have and understand how to use it to its full potential.
We must be prepared to make mistakes and to do it quickly in order to learn from our mistakes and not lose sight of the intermediate and final goals.
We are not alone.
We have this extraordinary group of people who value networking, exchange, and collaboration. A community of experts who can help us not waste resources and find the key to development in confrontation.
I look forward to our future discussions almost as much as to our future forests.
Books referenced in postcard illustration (left to right):
- Buijsman, S. (2021). Ada und die Algorithmen: Wahre Geschichten aus der Welt der künstlichen Intelligenz
- Thakur, A. (2020). Approaching (Almost) Any Machine Learning Problem
- Dauvergne, P. (2020). AI in the Wild: Sustainability in the Age of Artificial Intelligence