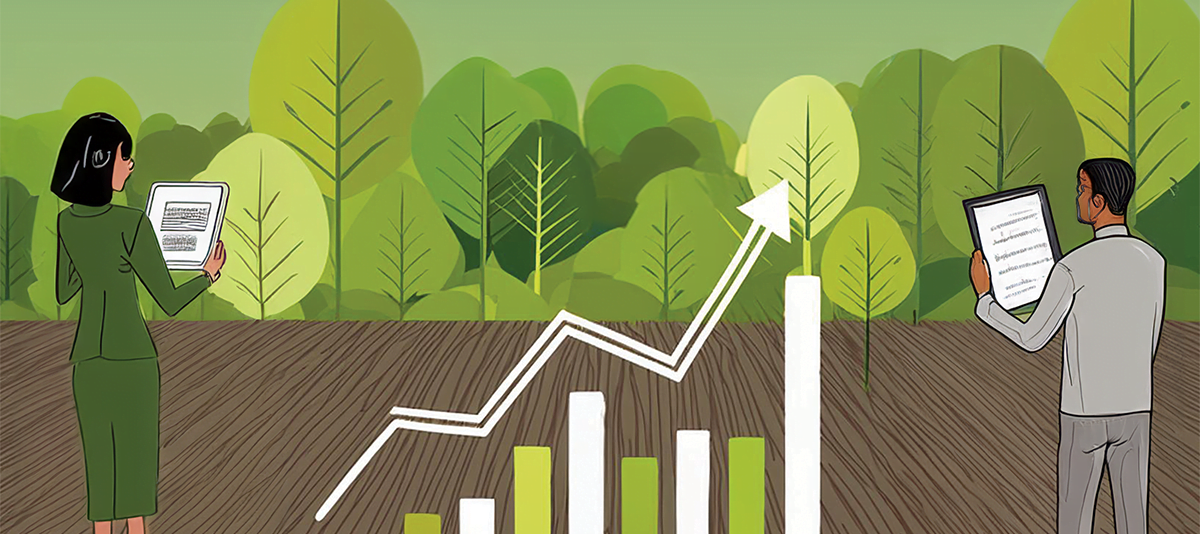
The Innovative Power of Annotated Data
Note: You can find the original German press release for this project here.
At present, the availability of high-quality annotated environmental data, usable to train Machine Learning (ML) algorithms is very limited. Within the project “LabelledGreenData4All”, the need as well as the potential of these high-quality data sets will be assessed. It further focuses on the development of an innovative data annotation process and green data spaces.
The Potential of Annotated Datasets
Considerable challenges are encountered when trying to combine environmental protection, digitalisation, and sustainability. Research and decision-making in these fields could be significantly fostered using Artificial Intelligence (AI). However, to do so, AI needs to have access to sufficient amounts of annotated data. Annotated data is data that has been tagged or labelled in a way that makes them understandable to ML-models. As such, properly annotated data sets form the foundation of effective machine learning and drive the further development of AI-supported environmental research.
The availability of suitable training data is crucial for the quality of the results ML models are able to provide. The effort required for data collection and processing is usually very high and is often repeated for individual model developments. The identification of application areas with high demand, the filling of data gaps, and the development of efficient annotation strategies are therefore essential for the successful and effective use of AI in the environmental sector.
The aim of LabelledGreenData4All is to develop strategic recommendations for the environmental sector regarding in which areas of application, and with which data, one can create the greatest potential applying ML models. It also addresses how to best support the exchange of annotated, or “labelled”, environmental data stemming from federal research.
“This research project should help us propose science-based political measures that will further promote and improve the exchange and collaborative usage of environmental data within data spaces across multiple sectors. As such, the project should not merely support the availability of annotated data for environmental applications, but also design the most sustainable process possible for annotating the data itself,” says Cathleen Mitzschke of Department Z 2.3 “Digital Transformation and Counselling Centre for Green IT“ at the German Environmental Agency (“Umweltbundesamt”, UBA).
Training Data is the Key – Development of an Innovative Process Model for Efficient and Scalable Data Annotation within the Environmental Sector
Existing annotation processes will have to be evaluated and analysed. In doing so, the project’s researchers need to pay particularly close attention to how scalable the solutions are, as well as the quality of their results. Based on this, a process model for data annotation will be developed, taking into account different data types and use cases.
“We want to develop an innovative process model that will enable efficient and scalable solutions for data annotation. This will allow the environmental sector to utilise AI-solutions both profitably and sustainably in the future,” explains Dr. Eva Klien of Fraunhofer IGD.
Data Spaces as a Digital Ecosystem
Improving the availability of annotated environmental and environmentally relevant data, as well as sharing such data across multiple sectors within green data spaces, is a central aspect of LabelledGreenData4All.
“Data spaces offer you the opportunity to make sensitive data available for a specific community, without having to sacrifice your control of said data. This is crucial, because currently only a maximum of about 40 percent of the data required for a specific use case consists of open data,” explains Thorsten Reitz, founder of wetransform.
By making such data more readily available, public authorities, researchers, and companies will be able to focus on innovation, rather than continuously having to expend the bulk of their development time on the gathering, preparation, and annotation of data.
The results from this research project should allow the environmental sector and other stakeholders within research, business/industry, and civil society to make better use of ML methods in the future. The potential of AI within the environmental sector needs to be explored and the technical gaps between its areas of application and the protection of the environment and resources closed. It is important to understand how AI can accelerate or hinder progress within sectors surrounding the climate and environment, as well as how these developments can be affected by various interested parties. The project therefore pursues an integrative research approach, with extra emphasis on the creation of networks between different communities and application-oriented transfer services.
We are assessing the current needs for annotated data for AI use cases related to environmental data and environmentally relevant data as well as climate. If you have already been involved in a project in which data was acquired and processed for the development of an AI model (in the broadest sense), we would highly appreciate it if you could fill out our questionnaire!